Data Continuity
Backup and recovery services are a necessity for todays modern networks. We can help to determine where and when your data needs to live to be sure it's always available
IT Consulting, Service and Management
Our decades of implementation and integration experience allows us to deliver best-of-class IT services to our customers
Cloud Services
With so many options and implementation scenarios available, let us help you determine how best to use new services available from the cloud.
Since 1996, our goal has been to help our clients maximize productivity and efficiency by expertly maintaining existing infrastructures, as well as designing and implementing new technologies, allowing them to continue growing into the future.
...
We focus on business process design and strategize and implement policies for continuous improvement and integration.
- Knowledgeable and friendly staff
- Flexible consumption-based pricing models
- Online strategy and consulting services
- Decades of experience
News, updates, trends and the latest
info you need to know about IT
April 30, 2024
Overview
The Intelligent Platform Management Interface (IPMI) implementations in multiple manufacturer’s Baseboard Management Controller (BMC) software are vulnerable to IPMI session hijacking. An attacker with access to the BMC network (with IPMI enabled) can abuse the lack of session integrity to hijack sessions and execute arbitrary IPMI commands on the BMC.
Description
IPMI is a computer interface specification that provides a low-level management capability independent of hardware, firmware, or operating system. IPMI is supported by many BMC manufacturers to allow for transparent access to hardware. IPMI also supports pre-boot capabilities of a computer such as selection of boot media and boot environment. BMCs are recommended to be accessible via dedicated internal networks to avoid risk of exposure.
IPMI sessions between a client and a BMC follow the RAKP key exchange protocol, as specified in the IPMI 2.0 specification. This involves a session ID and a BMC random number to uniquely identify an IPMI session. The security researcher, who wishes to remain anonymous, has attempted to disclose two vulnerabilities related to BMC software and session management. The first vulnerability identifies the use of weak randomization while interacting with a BMC using IPMI sessions. The researcher discovered that if both the IPMI session ID and BMC’s random number are predictable or constant, an attacker can either hijack a session or replay a session without knowing the password that was set to protect the BMC. The second vulnerability from the reporter identifies certain cases where the BMC software fails to enforce previously negotiated IPMI 2.0 session parameters, allowing an attacker to either downgrade or disable session verification. Due to the reuse of software or libraries, these vulnerabilities may be present in multiple models of BMC. It is recommended that sufficient precaution is taken in protecting datacenters and cloud installations with multiple servers to protect IPMI session interaction using both the software updates and the recommendations to secure and isolate the networks where IPMI is accessible.
Impact
An unauthenticated attacker with access to the BMC network can predict IPMI session IDs and/or BMC random numbers to replay a previous session or hijack an IPMI session. This can allow the attacker to inject arbitrary commands into the BMC and be able to perform high-privileged functions (reboot, power-off, re-image of the machine) that are available to the BMC.
Solution
Apply an update
Please consult the Vendor Information section for information provided by BMC vendors to address these vulnerabilities.
Restrict access
As a general good security practice, only allow connections from trusted hosts and networks to the BMC network that exposes the IPMI enabled interface.
Acknowledgements
Thanks to the security researcher who would like to remain anonymous for researching and reporting these vulnerabilities.
This document was written by Ben Koo.
April 29, 2024
Overview
A vulnerability in the R language that allows for arbitrary code to be executed directly after the deserialization of untrusted data has been discovered. This vulnerability can be exploited through RDS (R Data Serialization) format files and .rdx files. An attacker can create malicious RDS or .rdx formatted files to execute arbitrary commands on the victim’s target device.
Description
R supports data serialization, which is the process of turning R objects and data into a format that can then be deserialized in another R session. This will provide a copy of the R objects from the original session.
The RDS format, which mainly comprises .rds files, is used to save and load serialized R objects. These objects are utilized to share states and transfer data sets across programs. They are not expected to run code when they are loaded by an R implementation unless prompted by the user. R Packages use .rdx files, which contain a list of offsets, lengths, and names, and are accompanied by a .rdb file, which is used to extract more information about those offsets. .rdx and .rdb files contain RDS formatted data within themselves. A .rds file functions similarly to a .rdx file but only allows for storing a single R object. When loading a .rds or .rdx file, the readRDS function is utilized. An R implementation using the readRDS function given that information will then read the offsets and load the data.
R supports lazy evaluation. This can be implemented through a type called Promise, which can be represented in the RDS format as PROMSXP. This type is used to manage expressions that are called and completed in a asynchronous manner when their associated values are needed to be used by the program. When constructing an unserialized object in this context from the RDS format, the Promise object will require three pieces of data. These are the value of the Promise, the expression, and the environment. This information is loaded by the eval function. The eval function in R takes an expression, in this case the Promise, and evaluates it within the environment specified.
The vulnerability occurs when the eval function evaluates a promise type that has an unevaluated value. The Promise expression will not be properly evaluated and will execute the expression when it is referenced in the program that contains it. A threat actor can include malicious code within a .rds or .rdx file that is referenced by an unevaluated value. When an R implemention loads a package that contains an .rds or .rdx file and the promise value is reached, it will execute the referenced code. This code is arbitrary and will be executed prior to any opportunity for the victim to explore and see what functions or objects are within the file loaded.
Impact
An attacker can create malicious .rds and .rdx files and use social engineering to distribute those files to execute arbitrary code on the victim’s device. Projects that use readRDS on untrusted files are also vulnerable to the attack. Attackers can also leverage system commands to access resources available to the application and exfiltrate data from any environment available to the application on the target device. The code in the malicious files can also be used to access adjacent resources such other computers/devices, devices in a cluster and shared documents/folders available to the application.
Solution
Apply Updates
R project has provided R Core Version 4.4.0, which addresses the vulnerability. R Core version 4.4.0 now restricts promises in the serialization stream so that they are not used for implementing lazy evaluation. Apply the update at your earliest convenience.
Secure or Sandbox RDS file usage
Protect and use untrusted/third-party .rds, rdb, and .rdx files either in Containers or in a Sandbox environment to prevent unexpected access to resources.
Acknowledgements
Thanks to the reporter, Kasimir Schulz and Kieran Evans of HiddenLayer for reporting this vulnerability. This document was written by Christopher Cullen.
April 16, 2024
Overview
Lambda Layers in third party TensorFlow-based Keras models allow attackers to inject arbitrary code into versions built prior to Keras 2.13 that may then unsafely run with the same permissions as the running application. For example, an attacker could use this feature to trojanize a popular model, save it, and redistribute it, tainting the supply chain of dependent AI/ML applications.
Description
TensorFlow is a widely-used open-source software library for building machine learning and artificial intelligence applications. The Keras framework, implemented in Python, is a high-level interface to TensorFlow that provides a wide variety of features for the design, training, validation and packaging of ML models. Keras provides an API for building neural networks from building blocks called Layers. One such Layer type is a Lambda layer that allows a developer to add arbitrary Python code to a model in the form of a lambda function (an anonymous, unnamed function). Using the Model.save() or save_model() method, a developer can then save a model that includes this code.
The Keras 2 documentation for the Model.load_model() method describes a mechanism for disallowing the loading of a native version 3 Keras model (.keras file) that includes a Lambda layer when setting safe_mode (documentation):
safe_mode: Boolean, whether to disallow unsafe lambda deserialization. When safe_mode=False, loading an object has the potential to trigger arbitrary code execution. This argument is only applicable to the TF-Keras v3 model format. Defaults to True.
This is the behavior of version 2.13 and later of the Keras API: an exception will be raised in a program that attempts to load a model with Lambda layers stored in version 3 of the format. This check, however, does not exist in the prior versions of the API. Nor is the check performed on models that have been stored using earlier versions of the Keras serialization format (i.e., v2 SavedModel, legacy H5).
This means systems incorporating older versions of the Keras code base prior to versions 2.13 may be susceptible to running arbitrary code when loading older versions of Tensorflow-based models.
Similarity to other frameworks with code injection vulnerabilities
The code injection vulnerability in the Keras 2 API is an example of a common security weakness in systems that provide a mechanism for packaging data together with code. For example, the security issues associated with the Pickle mechanism in the standard Python library are well documented, and arise because the Pickle format includes a mechanism for serializing code inline with its data.
Explicit versus implicit security policy
The TensorFlow security documentation at https://github.com/tensorflow/tensorflow/blob/master/SECURITY.md) includes a specific warning about the fact that models are not just data, and makes a statement about the expectations of developers in the TensorFlow development community:
Since models are practically programs that TensorFlow executes, using untrusted models or graphs is equivalent to running untrusted code. (emphasis in earlier version)
The implications of that statement are not necessarily widely understood by all developers of TensorFlow-based systems.The last few years has seen rapid growth in the community of developers building AI/ML-based systems, and publishing pretrained models through community hubs like huggingface (https://huggingface.co/) and kaggle (https://www.kaggle.com). It is not clear that all members of this new community understand the potential risk posed by a third-party model, and may (incorrectly) trust that a model loaded using a trusted library should only execute code that is included in that library. Moreover, a user may also assume that a pretrained model, once loaded, will only execute included code whose purpose is to compute a prediction and not exhibit any side effects outside of those required for those calculations (e.g., that a model will not include code to communicate with a network).
To the degree possible, AI/ML framework developers and model distributors should strive to align the explicit security policy and the corresponding implementation to be consistent with the implicit security policy implied by these assumptions.
Impact
Loading third-party models built using Keras could result in arbitrary untrusted code running at the privilege level of the ML application environment.
Solution
Upgrade to Keras 2.13 or later. When loading models, ensure the safe_mode parameter is not set to False (per https://keras.io/api/models/model_saving_apis/model_saving_and_loading, it is True by default). Note: An upgrade of Keras may require dependencies upgrade, learn more at https://keras.io/getting_started/
If running pre-2.13 applications in a sandbox, ensure no assets of value are in scope of the running application to minimize potential for data exfiltration.
Advice for Model Users
Model users should only use models developed and distributed by trusted sources, and should always verify the behavior of models before deployment. They should follow the same development and deployment best practices to applications that integrate ML models as they would to any application incorporating any third party component. Developers should upgrade to the latest versions of the Keras package practical (v2.13+ or v3.0+), and use version 3 of the Keras serialization format to both load third-party models and save any subsequent modifications.
Advice for Model Aggregators
Model aggregators should distribute models based on the latest, safe model formats when possible, and should incorporate scanning and introspection features to identify models that include unsafe-to-deserialize features and either to prevent them from being uploaded, or flag them so that model users can perform additional due diligence.
Advice for Model Creators
Model creators should upgrade to the latest versions of the Keras package (v2.13+ or v3.0+). They should avoid the use of unsafe-to-deserialize features in order to avoid the inadvertent introduction of security vulnerabilities, and to encourage the adoption of standards that are less susceptible to exploitation by malicious actors. Model creators should save models using the latest version of formats (Keras v3 in the case of the Keras package), and, when possible, give preference to formats that disallow the serialization of models that include arbitrary code (i.e., code that the user has not explicitly imported into the environment). Model developers should re-use third-party base models with care, only building on models from trusted sources.
General Advice for Framework Developers
AI/ML-framework developers should avoid the use of naïve language-native serialization facilities (e.g., the Python pickle package has well-established security weaknesses, and should not be used in sensitive applications).
In cases where it’s desirable to include a mechanism for embedding code, restrict the code that can be executed by, for example:
disallow certain language features (e.g., exec)
explicitly allow only a “safe” language subset
provide a sandboxing mechanism (e.g., to prevent network access) to minimize potential threats.
Acknowledgements
This document was written by Jeffrey Havrilla, Allen Householder, Andrew Kompanek, and Ben Koo.
Contact us today if you'd like to know more
about how we can keep your network working at its best
VistaNet, Inc is a technology consulting and services company, helping enterprises
marry scale with agility to achieve competitive advantage.
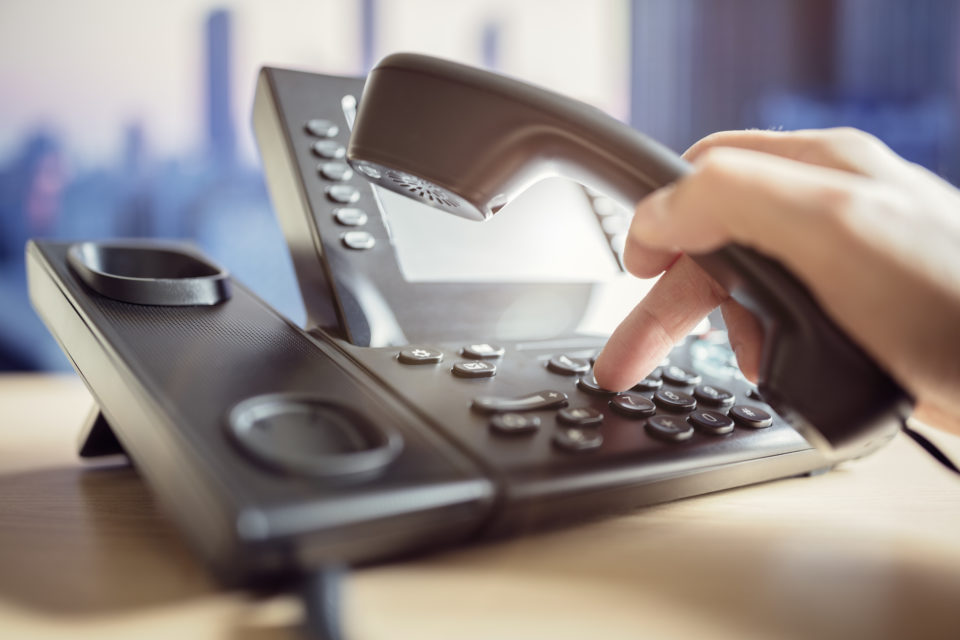